1. The Data Dilemma in Generative AI
Generative AI refers to a subset of artificial intelligence that creates new content—images, text, audio, video, and more—based on patterns it learns from existing data, raising questions about what challenge it faces with respect to data. Tools like ChatGPT, DALL·E, and Stable Diffusion are popular examples that showcase the potential of generative AI across industries. However, this impressive technology comes with a critical dependency: high-quality, large-scale datasets.
To perform well, generative AI models must be trained on vast amounts of data that are not only clean and diverse but also ethically sourced. This creates a significant tension between innovation and responsibility. Poor data quality can lead to misleading results; biased datasets can reinforce harmful stereotypes; and unregulated use of personal data can spark privacy concerns.
In short, the very fuel that powers generative AI also poses its greatest vulnerabilities.
Understanding what challenge does generative AI face with respect to data is essential for building trustworthy AI systems. This blog explores those challenges in depth—from data bias and privacy to data provenance—and also presents a forward-thinking solution: Generate Person by PiktID. This tool helps users protect identities in datasets by replacing or anonymizing people in images without compromising realism.
2. What Challenge Does Generative AI Face with Respect to Data?
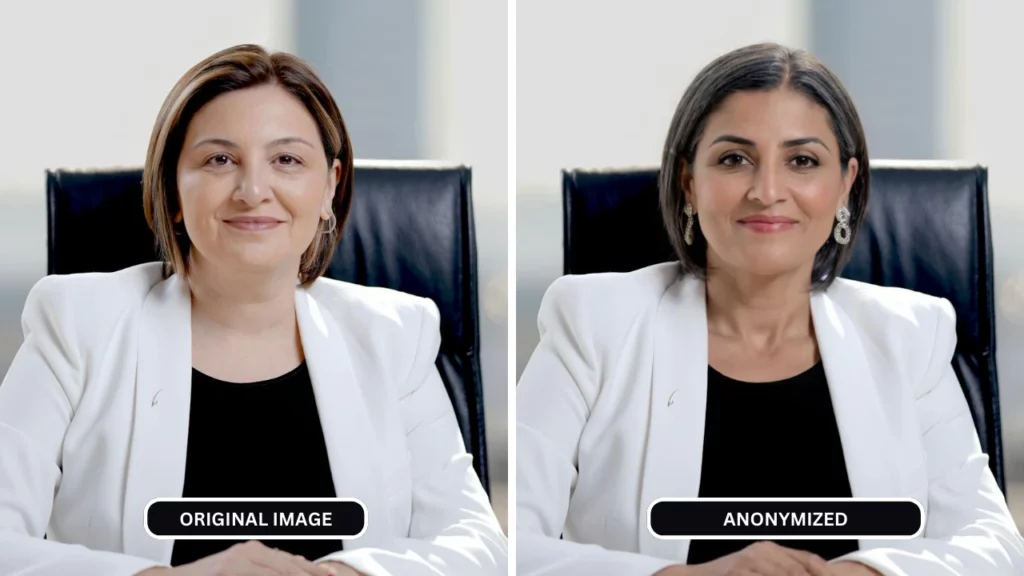
What challenge does generative AI face with respect to data?
“Generative AI faces major data-related challenges such as poor data quality, inherent biases, lack of data privacy, and unclear data provenance. These issues can result in flawed content, ethical risks, and reduced public trust in AI systems.”
One of the biggest challenges in generative AI is ensuring data quality. If a model is trained on noisy, mislabeled, or incomplete datasets, the results will be inaccurate or nonsensical. For example, an AI trained on poorly labeled facial images might generate distorted outputs when asked to create new faces.
Then comes bias—a pervasive issue that arises when training data overrepresents or underrepresents certain groups. A classic case involves resume filtering AI trained mostly on male applicants, which resulted in discriminatory hiring suggestions. Similarly, image-generation models have shown tendencies to favor certain racial or gender appearances, reinforcing stereotypes instead of challenging them.
Privacy is another cornerstone concern. Generative models can sometimes memorize parts of their training data and unintentionally reproduce personal information, such as faces or names. This raises serious ethical questions about whether people’s data—especially from publicly scraped websites—was ever meant to be used for AI training.
Lastly, data provenance, or the traceability of where data comes from, is often unclear. AI developers may not always know whether the data they’re using is ethically sourced, properly licensed, or even legally usable. This creates legal and reputational risks, especially for commercial applications.
When evaluating what challenge does generative AI face with respect to data, it’s clear that these aren’t just technical hurdles—they’re fundamental questions about ethics, ownership, and accountability in AI development.
3. Data Quality: Garbage In, Garbage Out
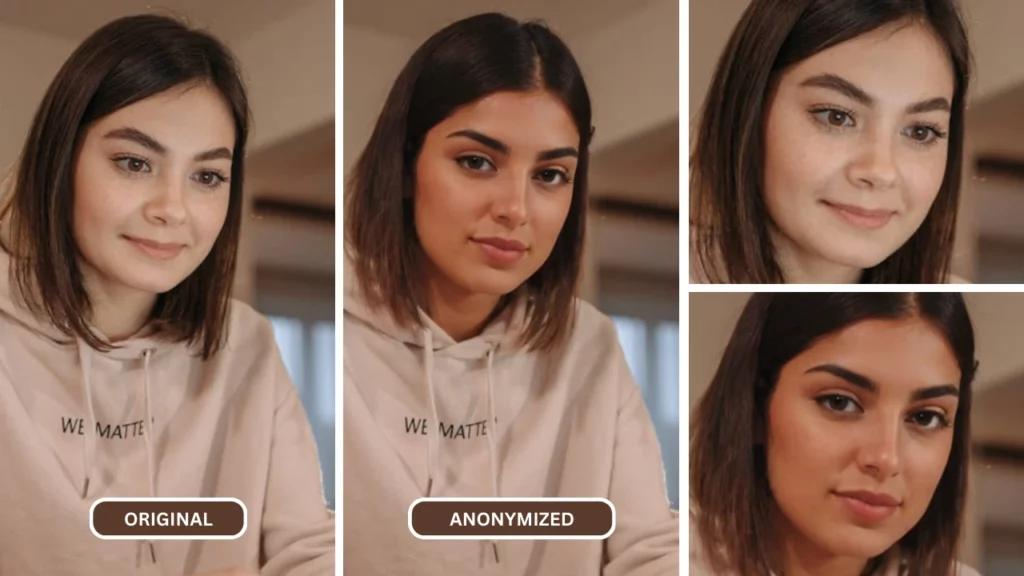
When asking what challenge does generative AI face with respect to data, one of the most pressing issues is data quality. Generative AI systems, whether they’re producing images, text, or audio, are only as good as the data they’re trained on. If that data is mislabeled, biased, incomplete, or of poor quality, the output will reflect those flaws—often in ways that are hard to detect until it’s too late.
Consider a text-generating model trained on outdated or inaccurate medical data. The AI might produce health advice that sounds plausible but is medically incorrect. In image generation, low-quality or poorly annotated images can lead to distorted faces, inaccurate skin tones, or offensive representations—especially in sensitive contexts like race or gender. For example, an AI trained with low-resolution celebrity images might hallucinate facial features when applied to real people. In audio, poor-quality training data can result in robotic or unintelligible speech synthesis that lacks the emotional nuance required for accessibility or virtual assistants.
This is particularly damaging in high-stakes fields like healthcare and finance. In healthcare, an AI-powered diagnostic tool trained on flawed data could misidentify tumors, leading to incorrect treatment. In finance, a generative model trained on skewed economic indicators might suggest unrealistic projections, harming investment decisions or client portfolios.
So in short, the “garbage in, garbage out” principle is a direct response to what challenge does generative AI face with respect to data: if the input data is broken, the entire AI pipeline suffers.
Read our in-depth Guide about AI fashion model generator for global campaigns
4. Privacy Concerns: Is Your Face in a Dataset?
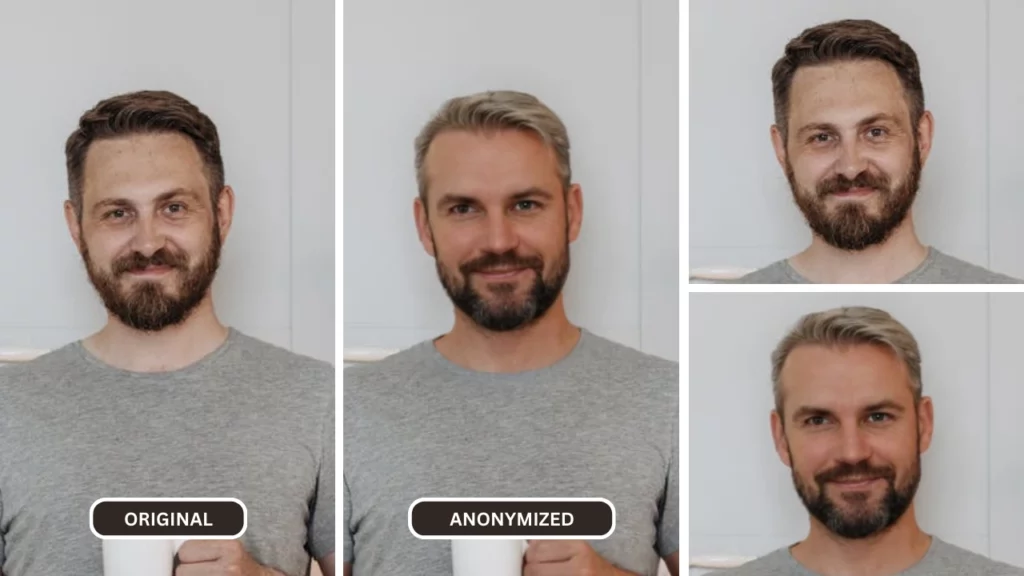
Another critical component in answering what challenge does generative AI face with respect to data is privacy—specifically, how easily AI systems can misuse or leak personally identifiable information (PII). Generative models, especially those dealing with facial recognition, voice cloning, and personalized content generation, often scrape massive volumes of publicly available data. The problem? Much of this data includes images and personal details that were never intended for training use.
For instance, someone might find their photo—originally shared on social media or a blog—repurposed by an AI to generate synthetic faces, deepfakes, or avatars without their consent. Even if the data was technically “public,” it wasn’t ethically sourced or permissioned for reuse. This grey area has triggered public backlash and legal scrutiny. In 2020, Clearview AI was sued for collecting billions of facial images from the web without consent, raising concerns about surveillance and data misuse.
Moreover, generative AI models can memorize and inadvertently reproduce sensitive data, such as names, addresses, and even biometric traits, if such data was present during training. This exposes users to identity theft, reputational harm, or unauthorized profiling.
When evaluating what challenge does generative AI face with respect to data, it becomes clear that data privacy is more than a legal formality—it’s a fundamental trust issue. Tools like Generate Person by PiktID help address this by allowing users to anonymize their images before they ever become part of a dataset, reducing risk while preserving creative freedom.
Additional Resource : Marketing tool face generator
5. Data Bias: Why Fairness Matters
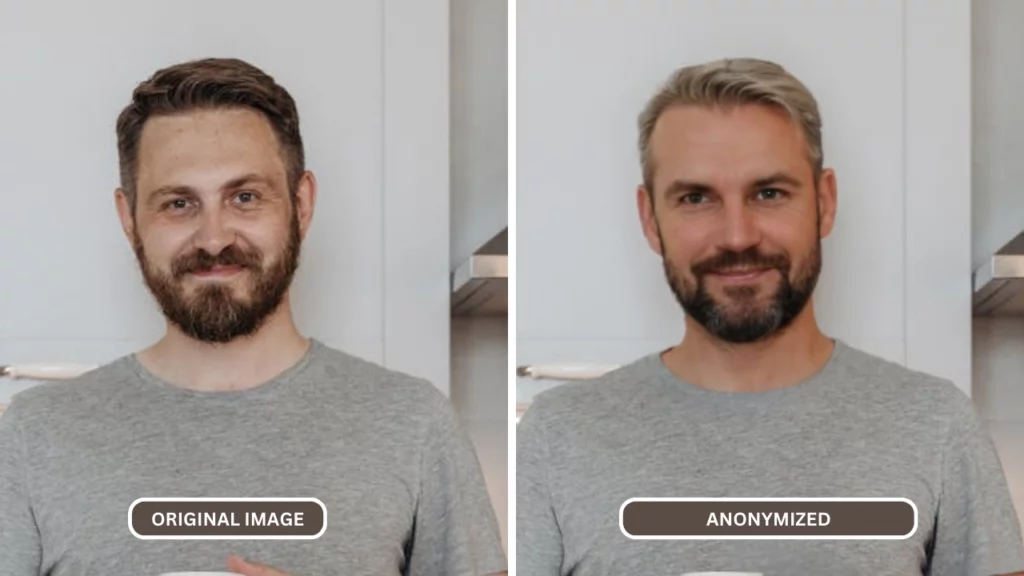
When asking what challenge does generative AI face with respect to data, one of the most critical issues is bias in datasets. Generative AI learns by identifying patterns in large volumes of data. But if that data is skewed—favoring certain demographics, tones, languages, or visual features—it results in biased outcomes that reinforce harmful stereotypes and lead to unfair decisions.
For instance, generative models used in AI hiring tools have shown gender and racial bias, often ranking male candidates higher than female ones for leadership roles. Similarly, facial recognition systems trained on imbalanced datasets have been found to misidentify individuals from minority groups far more frequently than white individuals. These discrepancies are not merely technical flaws—they directly impact real people by limiting job access, increasing surveillance risks, and perpetuating inequality.
The core challenge lies in balancing inclusivity with representational data. On one hand, AI developers must ensure all communities are fairly represented. On the other, they must respect data privacy and avoid over-sampling sensitive populations. When we ask what challenge does generative AI face with respect to data, addressing embedded societal bias is at the top of the list—because biased data produces biased machines.
6. Data Provenance: Where Did the Data Come From?
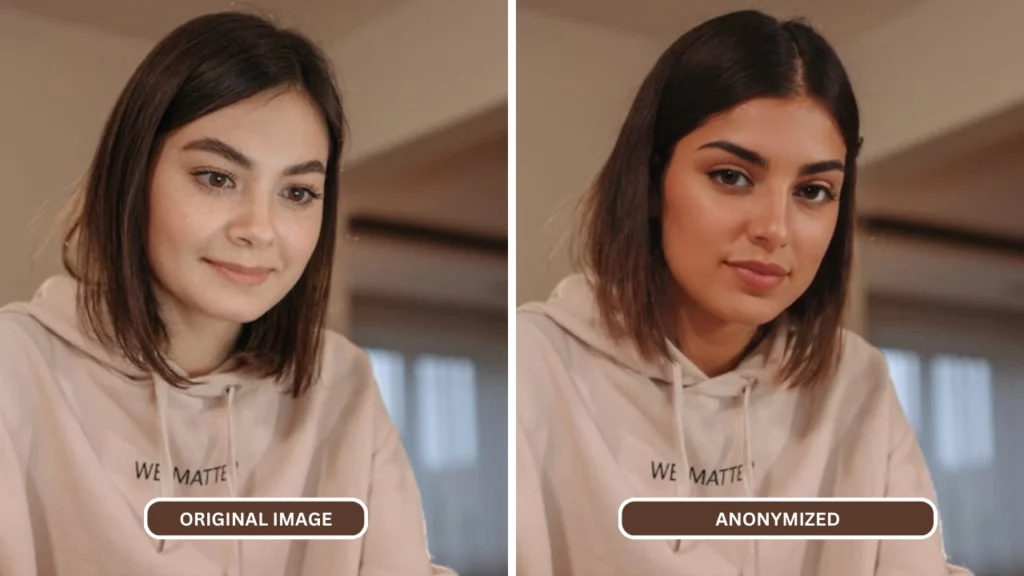
Another major concern in answering what challenge does generative AI face with respect to data is the issue of data provenance—the ability to trace the origin, licensing, and journey of training datasets. In simple terms, provenance is the “paper trail” of where data came from, how it was collected, and whether it was ethically sourced.
This becomes particularly critical when generative AI is trained on scraped data from websites, social platforms, or academic repositories, often without the knowledge or consent of the individuals whose data is being used. Not only does this pose ethical challenges, but it can also trigger legal consequences—especially when copyrighted or personally identifiable content is involved.
One of the core issues is the lack of transparency in many training datasets. Developers may use third-party sources that themselves lack proper documentation, making it impossible to verify whether the data was sourced legally or ethically. This undermines trust in generative AI systems and exposes companies to regulatory scrutiny.
When considering what challenge does generative AI face enhancer with respect to data, data provenance stands out as a foundational concern. Without clear sourcing and consent, the integrity of the entire AI model is compromised. For ethical, legal, and performance reasons, every piece of data used to train AI must be traceable, documented, and aligned with user rights.
7. Can Generative AI Work Without Large Datasets?
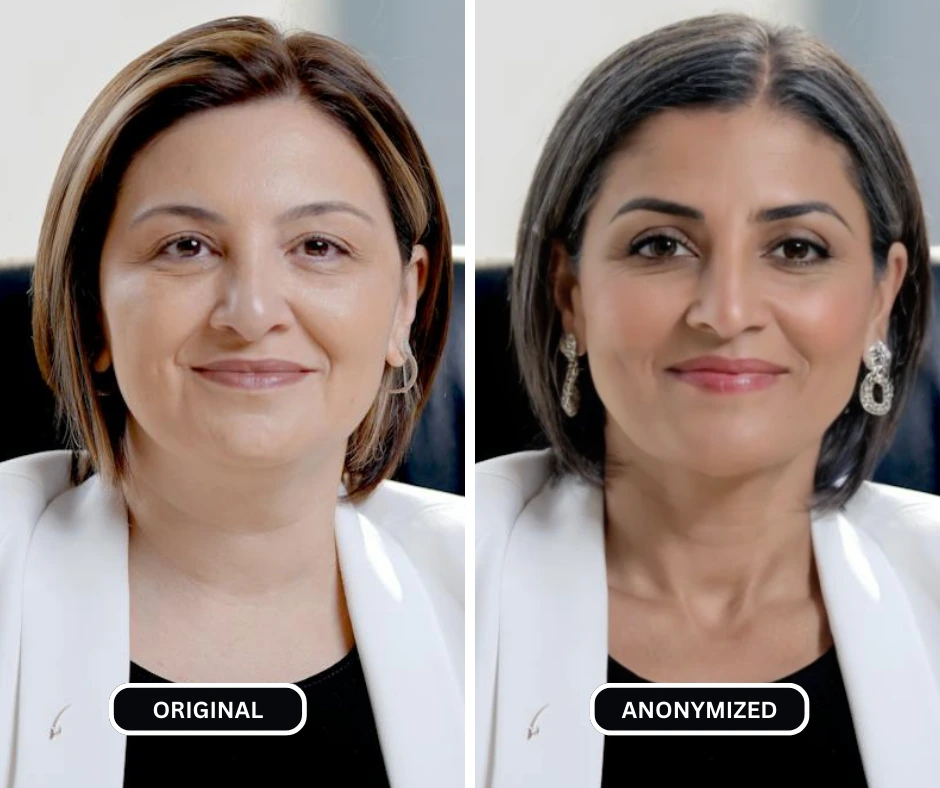
One of the most pressing questions in the world of machine learning is: Can generative AI truly work without massive amounts of data? The short answer is—yes, but not without compromises.
Techniques like few-shot learning and zero-shot learning have emerged as alternatives to traditional data-heavy approaches. These methods enable models to perform tasks with limited or even zero direct examples by leveraging pre-trained knowledge and adapting quickly to new scenarios. While promising, they still rely on vast pretrained models that were initially trained on massive datasets. So, the challenge isn’t entirely avoided—just shifted upstream.
This brings us back to a key issue: what challenge does generative AI face with respect to data? The answer lies in balancing innovation with responsibility. Even advanced techniques cannot completely eliminate the need for diverse, ethically sourced, and high-quality datasets. Without them, outputs risk being inaccurate, biased, or even harmful.
Smaller datasets, especially if skewed or poorly labeled, can limit the model’s generalizability and introduce hidden biases. Moreover, the reliance on publicly scraped or unverified data can lead to privacy violations and legal concerns. Hence, responsible data sourcing and anonymization tools become essential.
8. Solution Spotlight: Anonymize Photos Using Generate Person by PiktID
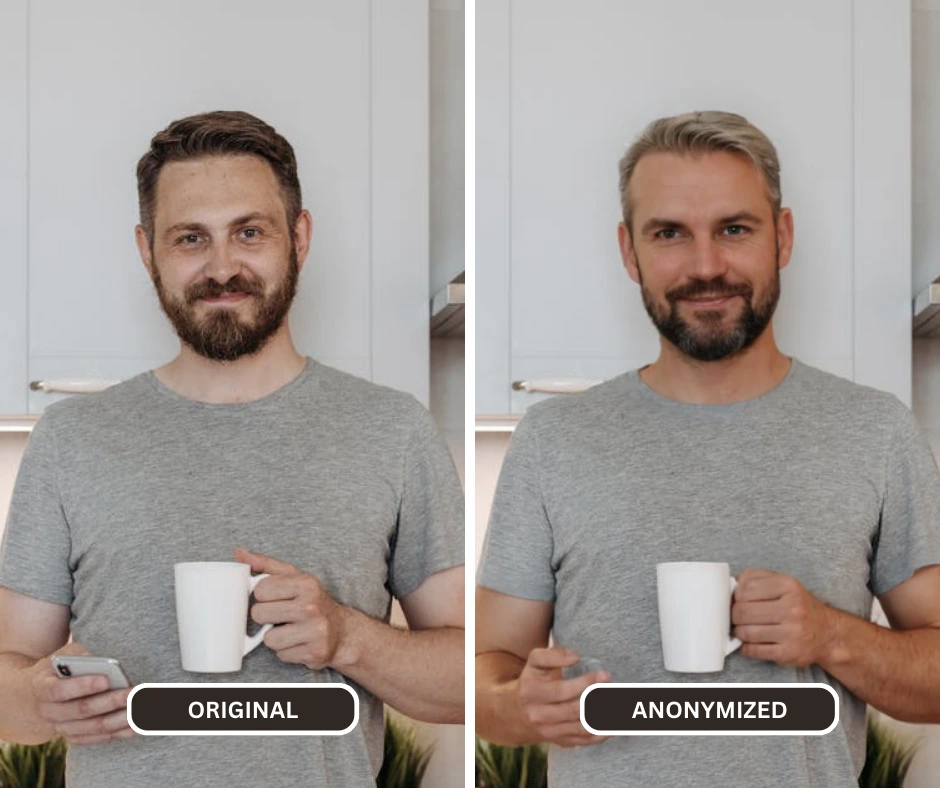
To address the privacy risks associated with training data, tools like Generate Person by PiktID offer a seamless way to anonymize visual data without compromising realism.
Whether you’re building datasets for research, marketing, user testing, or AI training, anonymizing personal data is no longer optional—it’s essential. One of the primary answers to what challenge does generative AI face with respect to data lies in tools that respect user privacy while enabling innovation.
Read More about EraseID Tutorial to learn and understand the steps involved in manipulating identity and facial expressions.
How to Use Generate Person by PiktID:
Step 1: Visit the Tool
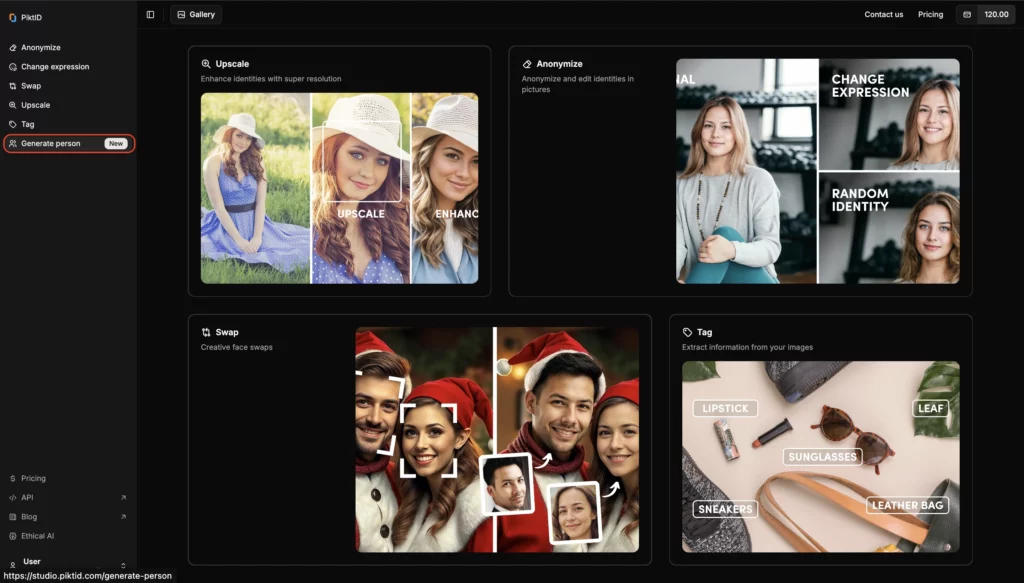
Step 2: Upload an Image
Drag and drop or select an image directly from your device. This can be a personal photo or any image you want to anonymize.
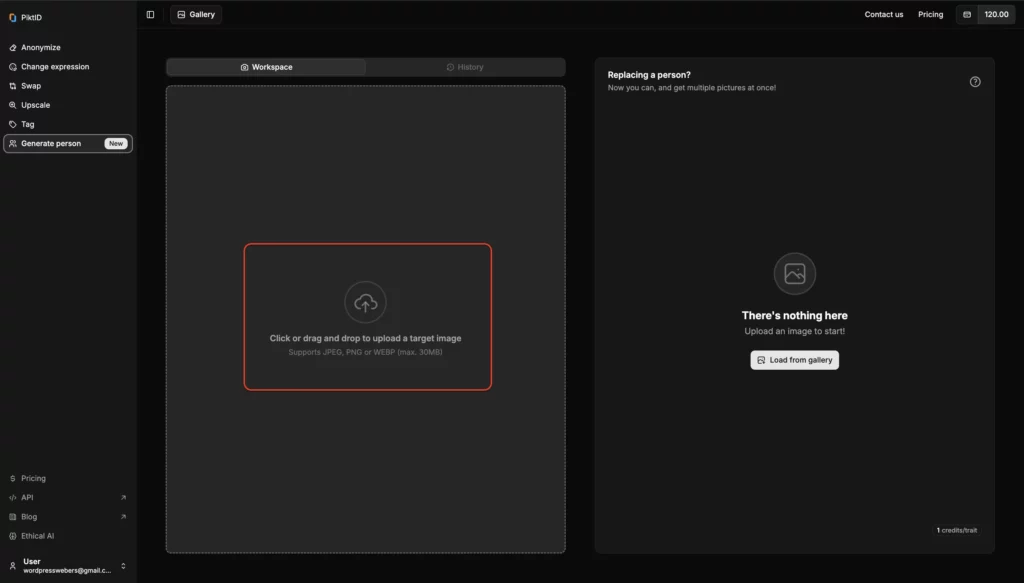
Step 3: Pick a Person to Replace or Clone
Using the right-side interface, select the person in the photo you’d like to anonymize. You can also clone or modify specific individuals.
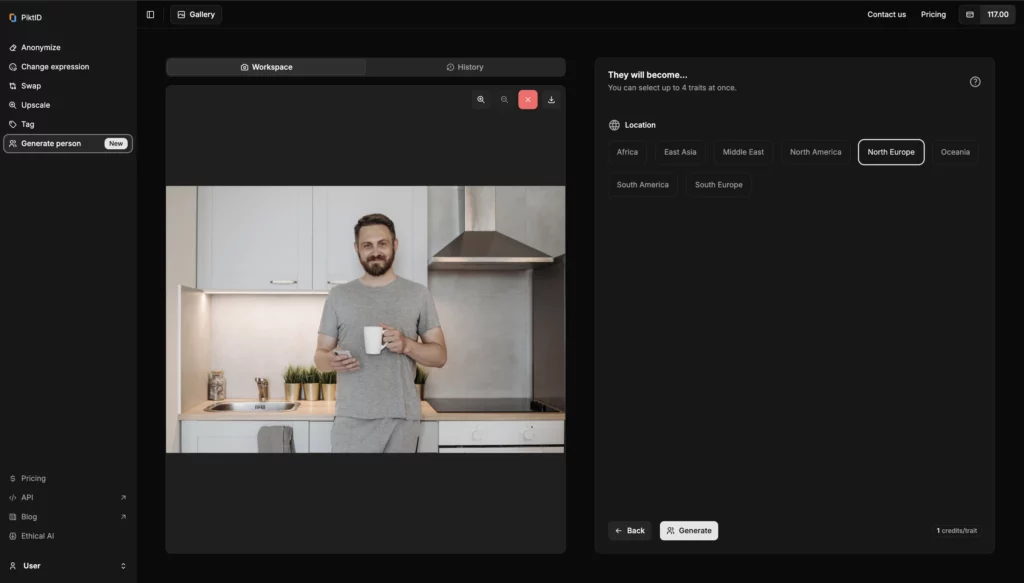
Step 4: Click Generate
Once selected, click the “Generate” button. The AI will process the image and create an anonymized version, which you can view in the History tab.
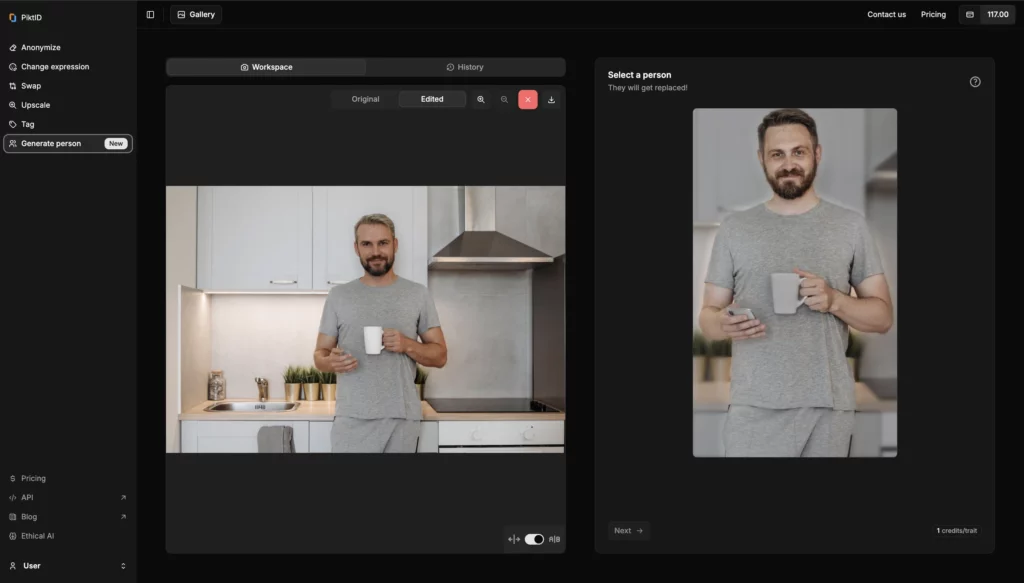
Benefits of Generate Person:
- Protects Privacy: Automatically replaces or alters identifiable faces, reducing the risk of misuse.
- Ideal for Dataset Creation: Create AI training datasets without exposing real individuals.
- Perfect for Marketing & UX Testing: Maintain human realism without breaching privacy laws.
- No Photoshop Needed: The AI handles all edits—no design skills required.
As privacy regulations like GDPR and CCPA tighten globally, tools like Generate Person solve a core issue in what challenge does generative AI face with respect to data—namely, data ethics, consent, and security. By anonymizing facial data at scale, you’re taking a proactive step toward responsible AI development.
9. Final Thoughts: Towards Ethical and Responsible AI
As we continue to explore the transformative potential of artificial intelligence, one critical question remains at the forefront: what challenge does generative AI face with respect to data? The answer lies in a complex web of issues—ranging from data privacy and security to bias, quality control, and provenance. These challenges are not just technical hurdles but ethical concerns that shape the trustworthiness and long-term viability of AI systems.
Without clean, diverse, and responsibly sourced data, even the most advanced generative AI models can produce outputs that are inaccurate, discriminatory, or invasive. That’s why developers, businesses, and individuals alike must prioritize ethical data practices.
This is where tools like Generate Person by PiktID play a vital role. By allowing users to anonymize faces in images while maintaining visual quality, the tool offers a practical solution to safeguard personal data without compromising functionality. It directly addresses one of the most pressing concerns in generative AI: the unauthorized use and storage of identifiable data.
In a world where AI is becoming increasingly visual and personal, users must also become more informed. Understanding what challenge generative AI faces with respect to data is the first step toward creating responsible digital spaces. Whether you’re building datasets, sharing images, or simply curious about how your data is used—being proactive is key.
Take a look at our guide on AI Generated ID by PiktID.
10. Claim Your Free Credits
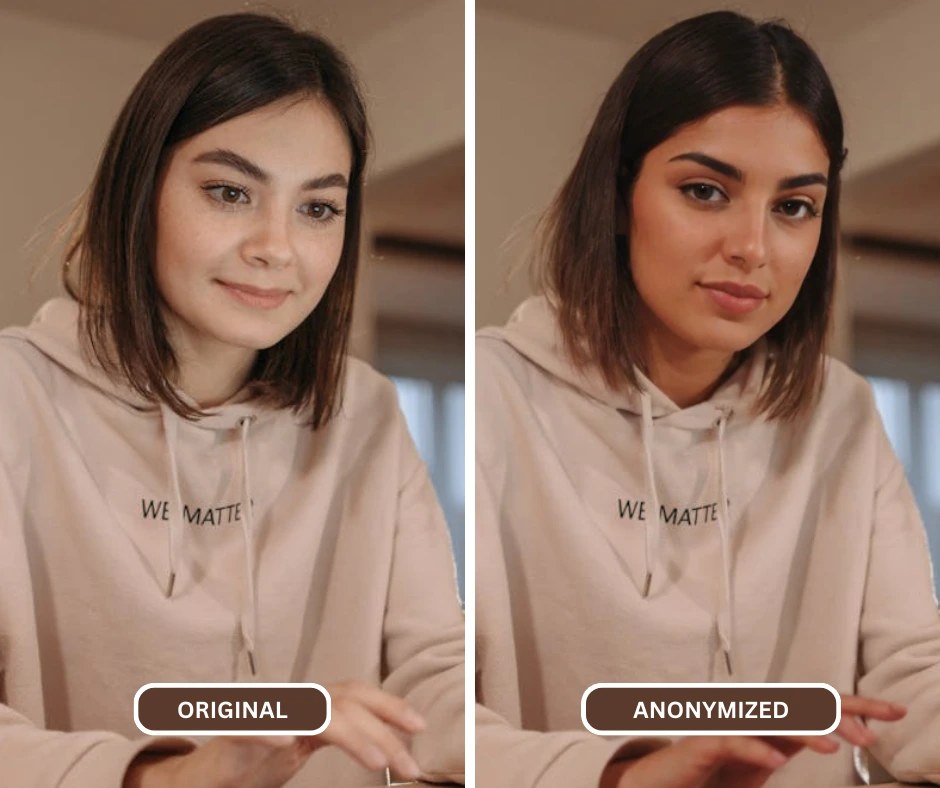
Start taking control of your privacy today with Generate Person by PiktID—a secure, user-friendly solution designed to anonymize facial data in seconds.
No downloads, no complications—just upload your photo, select the face to be changed, and hit generate. In just a few clicks, your image is ready—privacy intact.
Get started now and enjoy 10 free credits, with no signup cost required. Explore the power of ethical AI tools that put user control and data dignity first.
11. FAQs: Common Questions About Generative AI and Data
Q1: What is the biggest challenge generative AI faces today?
The biggest challenge is balancing data quality, bias, and privacy. Without clean, diverse, and ethically sourced data, generative AI can produce inaccurate or harmful results.
Q2: Can I prevent my data from being used in AI training?
Yes, by using privacy-focused tools like Generate Person by PiktID, you can anonymize your photos. These tools ensure that your data isn’t stored, reused, or added to training datasets.
Q3: Why is bias in AI training dangerous?
Bias in training data can lead to unfair, discriminatory, or inaccurate outputs. This is especially harmful in areas like facial recognition, hiring, lending, and healthcare where fairness is critical.
Q4: What makes a dataset high quality for generative AI?
A high-quality dataset is diverse, well-labeled, ethically sourced, and free from noise or duplication. It should represent real-world variations without reinforcing stereotypes or excluding key groups.
Q5: Is my face safe when I upload it to an AI tool?
It depends on the platform. Tools like Generate Person by PiktID are privacy-first—they do not store, reuse, or share your photos. Always choose platforms with clear, transparent data policies.
Q6: Can generative AI work without big datasets?
Not effectively. While few-shot and zero-shot learning techniques exist, generative AI performs best with large, diverse datasets. These models need variety to generate accurate and useful content.
Q7: How does generative AI use personal data?
Generative AI may retain traces of personal data from training sets, even unintentionally. If the data isn’t anonymized or consented, it can result in privacy risks and unauthorized replication of personal information.
Q8: What is data provenance, and why does it matter in AI?
Data provenance refers to tracking where data comes from and how it’s been used. It’s essential for ethical AI development, helping ensure transparency, legality, and accountability in training models.